Demystifying the Correlation Conundrum
- Sunil Kappal
- Jan 31, 2018
- 2 min read
It often gets confusing and terrifying when trying to solve the puzzle of which correlation technique should be deployed based on the X and Y variables properties. In this article we will discuss two highly used Correlation Analysis Techniques i.e. Pearson Correlation Coefficient and Spearman Rank Correlation.
Correlation is a bivariate technique that measures the relationship strength between two variables. The value of correlation varies between +1 & -1, where +1 denotes a highly positive relationship in between two variables and -1 indicates the inverse. As the correlation coefficient value goes near 0, the relationship between the two variables tends to get weaker.
Pearson Correlation
This correlation technique is widely used in statistics to measure the strength of the relationship between linear related variables. For example, in the contact center, if we want to measure how two metrics are related to each other (Call Duration and Non-Talk), Pearson correlation technique can be used to measure the degree of relationship between these two variables.
The following formula is used to calculate the Pearson correlation:
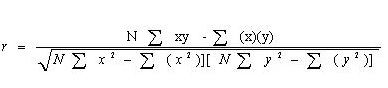
R = Pearson r correlation coefficient
N = number of value in each data set
∑xy = sum of the products of paired scores
∑x = sum of x scores
∑y = sum of y scores
∑x2 = sum of squared x scores
∑y2 = sum of squared y scores
Questions that can be answered by Pearson correlation
Is there a statistically significant relationship between call duration and Non-Talk?
Is there a relationship between temperature, measure in degree Celsius and ice cream sales, measure by income?
Is there a relationship between age in years and height in inches?
Assumptions
Pearson correlation technique assumes that both the variables are normally distributed. It also assumes that there is a linearity and homoscedasticity in between the variables. Linearity assumes a straight line relationship between each of the variable and homoscedasticity assumes the normally distribution along the regression line.
Spearman Rank Correlation
Spearman rank correlation is a non-parametric test which is used to measure the degree of association in between the two variables. It was developed by Spearman, thus called the Spearman rank correlation. Spearman test assume anything about distribution of the data. It is appropriate to use the Spearman rank correlation test when the variables are measured on a scale that is ordinal.
The following formula is used to calculate the Spearman rank correlation:
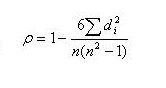
Where:
P = Spearman rank correlation
di = the difference between the ranks of corresponding values Xi and Yi
n = number of value in each data set
Questions that can be answered by Pearson correlation
Is there a statistically significant relationship between participant responses to two Likert scales questions?
Is there a statistically significant relationship between how the Member Experience Surveys are done on the scale of 1 – 10 compared to their experience scores?
Assumptions
Spearman rank correlation test doesn’t make any distributional assumptions about the data. The assumptions of Spearman rho correlation are that data must be at least ordinal and scores on one variable must be monotonically related to the other variable.
コメント